A deep understanding of the communities relevant to centers and the continual optimization of customer experience have become the mantra of the Placemaking Industry. Heterogeneous lifestyles in close proximity, sometimes conflicting lifestyles, and diverse brand affinities are now the success- defining benchmarks for the unique character and future orientation of each center. However, the necessary market adjustments come with substantial investments.
Decades-long used statistics and derived industry metrics are gradually losing their significance as they no longer realistically depict the complexities of the market. The demands on risk assessment, built on substantial and current market information, are accordingly high. With increasing industry-specific applications of AI, a glimmer of hope seems to appear, offering all answers and solutions for an optimized market approach in the catchment area of centers almost at the push of a button. That, of course, is compatible with existing location evaluation and analysis formats. If that were the case, would it be enough to successfully implement the necessary market adjustments?
As of today, AI can analyze existing, but hidden, market knowledge in the context of posed questions and incorporate it into market models, helping to significantly minimize decision and investment risks. Practically proven AI-supported analysis methods provide profound qualitative insights into existing life worlds. The gathered data, due to its density and sample size, is quantifiable, thus portraying real and market relevant communities. That creates orientation and helps substantially categorize sustainable developments in the catchment areas of centers.
The innovative and multi-award-winning analysis method of evAI recognizes relevant communities and their life styles, affine brand worlds, market participants, barriers, as well as opportunities, risks, and disruptive forces. From the acquired data, evAI models forward-looking market models and identifies existing and potential catchment areas. Practical questions (exemplary excerpt) are answered by evAI:
- How is the location environment structured and integrated into existing and future traffic flows?
- Which communities can be identified in the catchment area?
- What lifestyles (brand usage, affinities, areas of interest) characterize these communities?
- Which brands, services, etc., align with these lifestyles and the respective location?
- What is the optimal offer mix for the location, ensuring increased footfall?
- How can the catchment area be expanded with an attractive offering?
- How does a location differentiate itself from the competition for existing and potential communities?
The data obtained through such AI-supported analysis methods and the insights gained from them are current, cover a representative observation period, and can be projected onto future developments of the location. To a certain extent, the applied analysis methods meet the criteria that the Placemaking Industry increasingly demands from data analytics and research.
Challenges for Resposible Center Management
However, the challenge for responsible center management begins, at the latest, with presenting the findings and concrete answers to posed questions: The recently obtained data bluntly exposes the blind spots and deficiencies of locations – deficiencies that could lead to critical inquiries from stakeholders – investors, owners, and tenants.
For example: A centrally located shopping center in a metropolitan region lies directly on highways connecting the surrounding areas with the city center – a crucial decision criterion for the location, coupled with the idea of “capturing” people on their way to the city center and ensuring increased footfall in the shopping center due to favorable transport connections. That consideration, combined with an investment in a slightly oversized parking structure, was coherent but did not lead to the desired success. The reason for that was shown by the traffic situation of the location analyzed via mobility and traffic data. The surrounding residential area is a well-shielded block, on the one hand, through trains and highways, and on the other hand, through regular traffic jams at the entrances during peak shopping times. Within the neighborhood, oneway streets and an almost complete lack of a traffic guidance system complicate finding the location. An AI-analyzed traffic study not only reveals such a circumstance, but leads to an individual traffic guidance system, guaranteeing almost congestion-free and time-saving access to the shopping center and optimizing the visibility of the shopping center within the immediate catchment area. However, implementation and optimization has not occurred thus far.
Analysis of Lifestyles
The analysis of lifestyles in dense urban areas and urban agglomerations is more complex: Housing shortages, rising rental prices, new working formats – co-working spaces, home offices, etc. – have led to lifestyles in neighborhoods colliding that were inconceivable or not sought after just a few years ago. Such developments cannot be deduced from existing purchasing power data related to a location’s environment. In other words, that means that my neighbor, who has nearly the same purchasing power as me, lives in a completely different world, has a different lifestyle, and consequently prefers completely different brands and offerings. It sounds trivial, but it is not.
With the help of innovative, AI-supported analysis methods, different life spaces can be identified on a very local level and evaluated in terms of brand affinities. Social genome data provides specific insights into these lifestyles and thus the market relevance of potential offerings and brands. In a specific case, a shopping center was able to attract an international sports retailer as a potential anchor tenant for a central and attractive location. The preceding standardized location analysis revealed that “sports” in the immediate catchment area was a relevant topic, but attractive providers offering a broad range in the immediate vicinity were lacking. Therefore, tenants and center management expected a longterm collaboration, successful market acceptance of the sports retailer, and a continuous increase in footfall at the shopping center. None of that happened as expected; the tenant terminated the lease, and the space is now vacant. The subsequent AI-supported analysis of social genome data confirmed that “sports” at that location was a very relevant topic, and there was a lack of brand affinity of the target groups in question to the original tenant’s brand, in contrast to other brands identified as the “best brand match” in the sports context for that location.
Brands with high Affinity for the targeted Audience
In general, a location benefits from brands that have a high affinity for the targeted audiences of the center. Conducting a “best brand match” analysis before deciding on an offer area, provider, or brand significantly reduces risk – for both center management and potential tenants.
The data collected with the support of AI makes it an essential factor that currently determines, and will continue to do so in the future, the success of a center at its location. Further dimensions of consideration depend heavily on the individual circumstances of the location. In other words, what applies to one location may only apply to a very limited extent or not transferred at all to other locations. That poses significant challenges for operators of department store chains and center brands. The same applies to retail chains, consumer markets, etc., that feature standardized portfolios of offerings. Each location is an individual entity due to its surroundings, requiring a separate examination.
The relevant catchment area for the respective location is no longer defined by geographical and sociodemographic features, but by a virtual map of lifestyles connected to the shopping source – like a nervous system through analyzed traffic flows and connections. This forward-looking perspective on locations requires a differentiated and individual examination of locations and their previous indicators and KPIs. First and foremost, it requires the courage of all players in the placemaking industry to think differently about existing properties and to take planned developments in new but evidence-based directions. The analysis process supported with AI is a structured process that is openly curated by experts along questions about the location of a center. Hence, fostering an environment of curiosity, openness, and the courage to explore uncharted territories is imperative for all stakeholders. This enables the emergence of unforeseen questions, some of which may not have been apparent at the initiation of the AI analysis process. Are you ready for it?
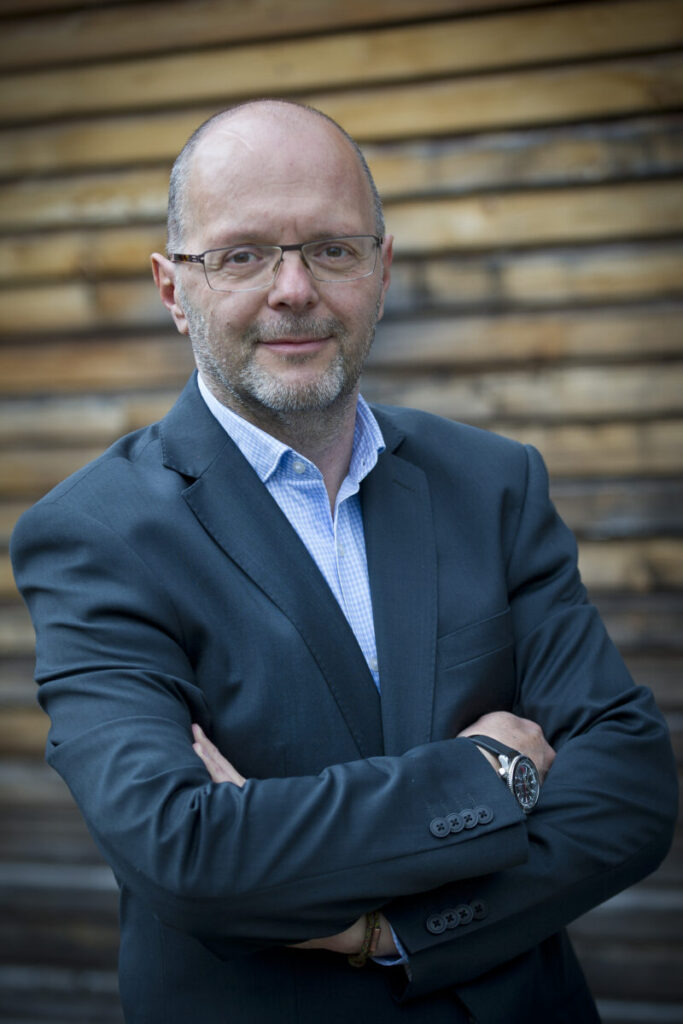
Oliver Zügel
Oliver Zügel, Chairman of GALLUP Swiss and a Board Member at evAI, specializes in business development and strategy.